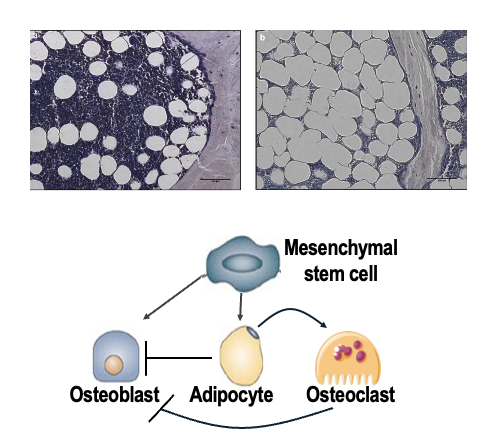
In individuals with type 2 diabetes (T2D), fracture risk is increased, in part due to intrinsic skeletal disease. Among the multifactorial etiologies of skeletal fragility in T2D, bone marrow adipose tissue (BMAT) is a metabolic fat depot that may also play a negative role in skeletal disease and specifically in T2D. Whether enhanced glycemic control affects skeletal outcomes through pathways such as BMAT is unknown.
Our group is currently investigating the effects of improved glycemic control on BMAT and skeletal outcomes in a longitudinal, prospective study of older Veterans with type 2 diabetes at the San Francisco VA. To date, 48 participants have enrolled and are actively working with their clinicians to improve the medical management of their diabetes. Eighty-eight percent of participants were men, the average age was 66 years, and the mean BMI was 32 k/m2. In our interim analyses, we observed a notable reduction in mean hemoglobin A1c levels from 9.4% to 7.9%, indicating a clinically significant improvement in glycemic control. We did not observe a statistically significant change in total vertebral BMAT content, assessed by magnetic resonance spectroscopy. However, mean femoral neck BMD declined by -1.4% ± 2.9% (p=0.006) and there was a negative correlation between changes in femoral neck BMD and total vertebral BMAT content (r=-0.45, p=0.01). Meaning participants who had greater declines in femoral neck BMD generally had increases in vertebral BMAT.
As we continue to collect and analyze data, we are excited to better understand underlying mechanisms that drive the relationship between glycemic control, BMAT, and skeletal health in people with T2D. These insights hold the potential to inform more targeted and effective clinical interventions for managing diabetic bone disease and mitigating the risk of fractures in this vulnerable population.
Assistant Professor of Medicine, Division of Endocrinology and Metabolism, UCSF & Staff Physician, San Francisco VA Health Care System
Research interests: osteoporosis, bone metabolism
Richard Souza, PhD, PT - The Relationship between Gait Biomechanics and Exercise Induced Pain Flares in Patellofemoral Joint Osteoarthritis
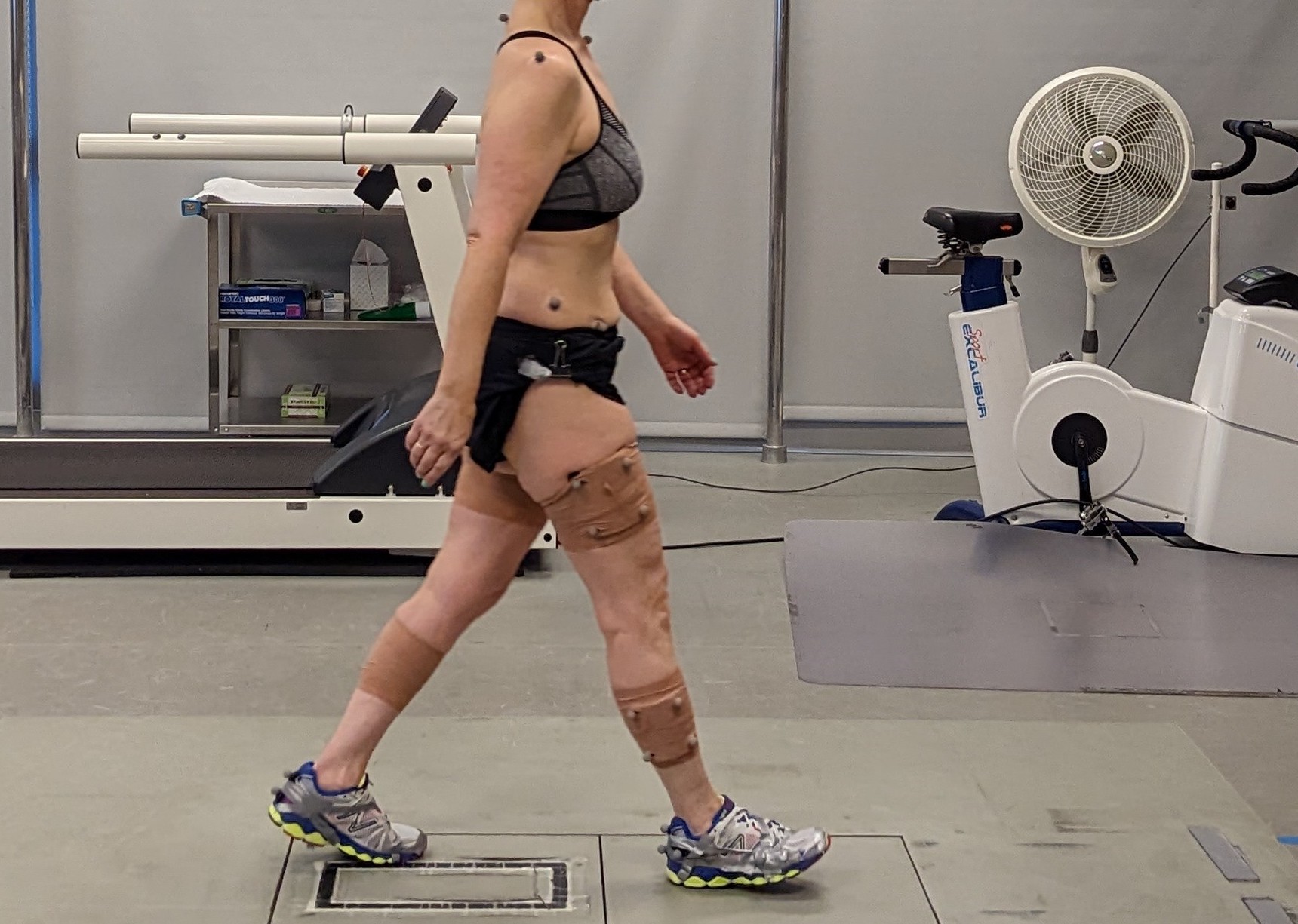
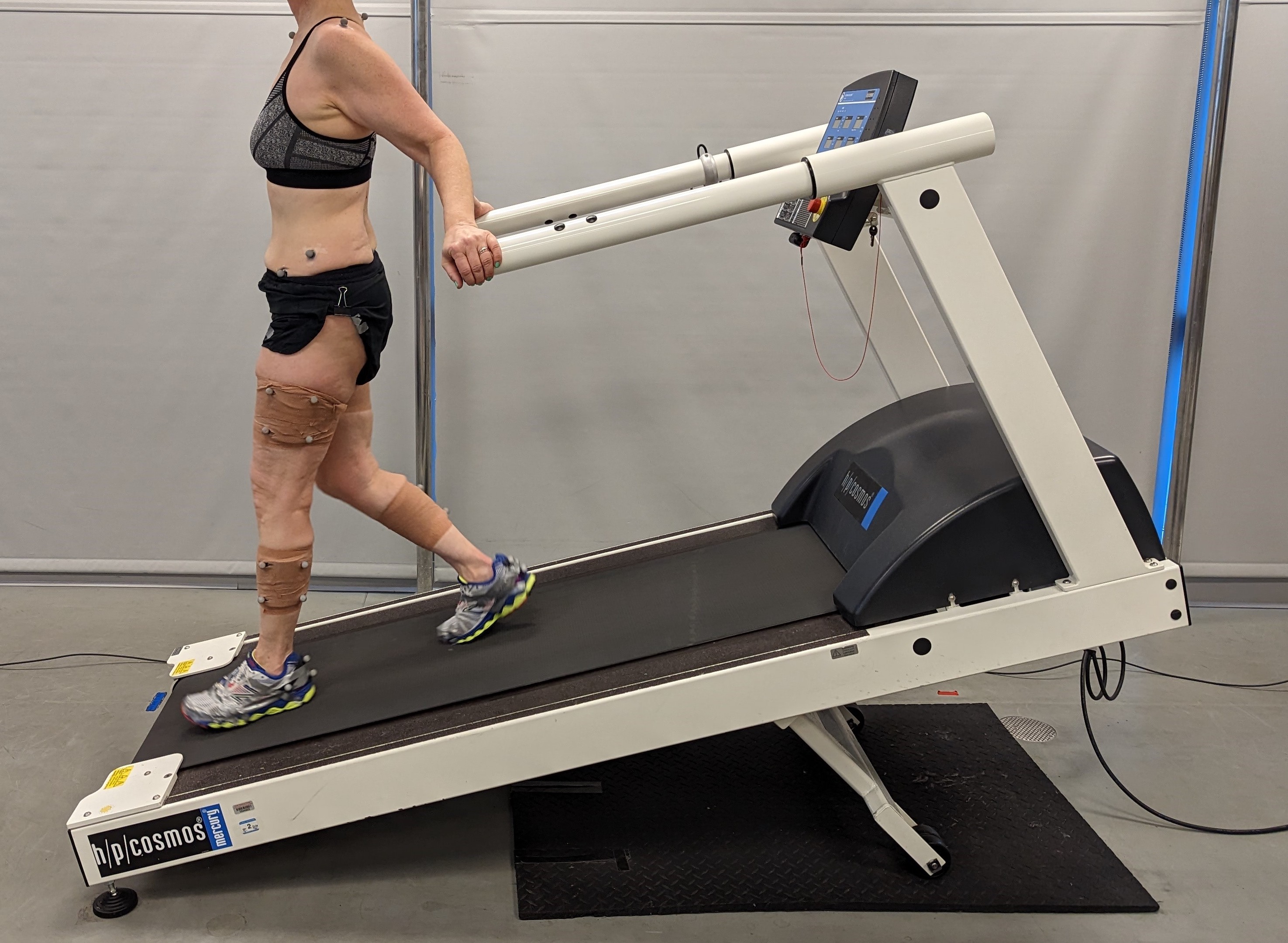
Based on radiographic and magnetic resonance imaging studies, 64% of adults over 50 years old have PFJ (patellofemoral joint) OA, with one-third of them having isolated PFJOA. Furthermore, PFJOA is a major source of pain and dysfunction. Joint loading is integral to OA progression yet currently, very little is known regarding the biomechanical factors associated with PFJOA progression. The overall objective of this work is to identify specific gait biomechanics that predispose individuals to pain flares, and to identify changes in gait biomechanics that result from increases in pain symptoms. We are conducting a cross-sectional, single cohort, interventional study to evaluate the role on pain flares on gait biomechanics. Each subject will complete gait and stair trials (ascend and descend) at the UCSF Human Performance Center while we collect 3D motion capture using a 6 degree-of-freedom cluster-based marker set. Joint loading will be calculated as knee moments using our imbedded force plates and standard inverse dynamics equations. Following baseline data collection, all subjects will complete a downhill walking protocol at 2.5 mile per hour (comfortable walking speed) with increasing decline from 10–25 % grade. Knee pain will be monitored every minute during the treadmill walking task. A clinically significant pain flare will be defined as increase in pain of 4 points (e.g. increase from 2/10 pain to 6/10 pain) or a maximum of 7 (e.g. if they started at 4/10 pain, they would qualify as a pain flare at 7/10) on the verbal Numerical Pain Rating Scale. Gait biomechanics for walking and stair climbing will be repeated identical to the baseline collection. We hypothesize that subjects that experience a significant increase in pain symptoms will demonstrate abnormal gait mechanics than those who don’t experience a pain flare. These data are critically necessary to understand the biomechanical mechanisms of pain production in subjects with PFJ OA. Currently there is a paucity of data on these subjects, and it is becoming increasingly clear that PFJ OA is the predominant initiation into knee OA in other compartments. As such, these data may have implications beyond isolated PFJ OA and may lead to additional intervention targets for patients suffering from painful OA.
Professor, Physical Therapy
Research interests: Injury mechanics, rehabilitation, lower extremity biomechanics, injury prevention, osteoarthritis, medical imaging, dynamic magnetic resonance imaging, motion analysis, quantitative imaging
Christine Hong, DMD, MS - Role of p75NTR in Osteogenic Differentiation and Skeletal Development using Mouse Model
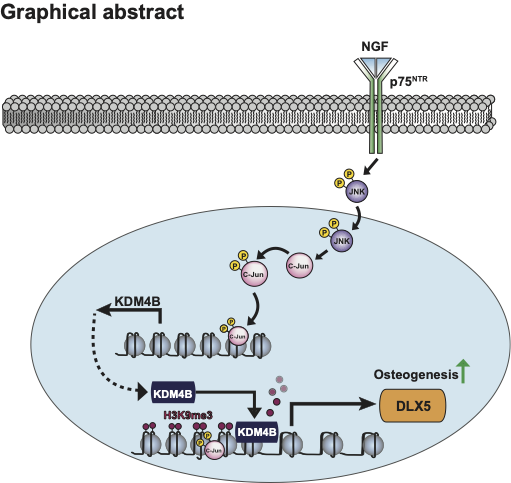
Figure 1: Molecular and epigenetic mechanism of NGF signaling in human mesenchymal stem cells during bone formation
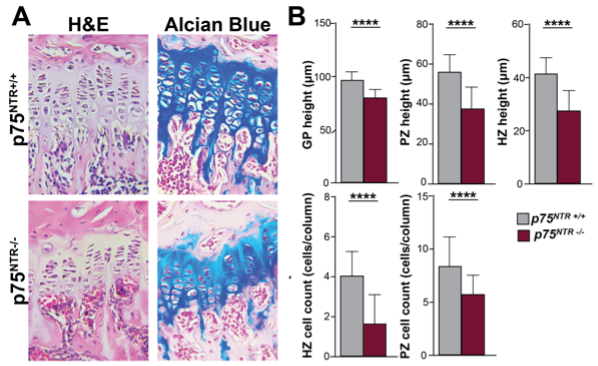
Figure 2: The loss of p75NTR leads to decreased rate of growth. (A) Representative epiphyseal plates from H&E staining & Alcian Blue staining at 4W (B) Quantification of heights and cell number in the columns of each zone (GP: growth plate, PZ: proliferative zone, HZ: hypertrophic zone) Data are presented as means ± SEMs (n=4-5). **** p<0.0001
Osteoporosis is a major public health concern acting as a leading cause of morbidity and mortality in our aging population and affecting over 200 million people worldwide. The financial burden from the costs of osteoporotic fractures is increasing at alarming rates. As current therapies for osteoporosis have numerous shortcomings, the development of new osteogenic therapies is imperative. Cell-mediated therapy using mesenchymal stem cells (MSCs) holds great promise for skeletal disorders. However, its reported clinical outcomes have been suboptimal, requiring a more precise understanding of the molecular and epigenetic regulatory mechanisms for MSC differentiation. NGF is recognized for its key role in the survival and maintenance of sympathetic and sensory neural networks. Recent findings revealed NGF actions in cells beyond neuronal cells and its participation in bone formation. NGF mediates through two receptors, TrkA and p75NTR. The role of TrkA in skeletal biology is increasingly elucidated. However, the role of p75NTR in bone remains largely unknown. Our mechanistic studies elucidated the critical role of the p75NTR/JNK/KDM4B regulatory axis in the epigenetic regulation of the NGF-dependent osteogenic response. In order to further examine the function of p75NTR in vivo, p75NTR was globally deleted and specifically deleted in multipotent mesenchyme. The loss of p75NTR led to decreased body weight, length, decreased growth plate thickness, and reduced bone formation for both whole body and conditional knockout mice. In this CCMBM Pilot/Feasibility proposal, we aim to use animals with the inducible Prx1-CreER to study the effect of p75NTR in postnatal skeletal development and growth. We hypothesize that p75NTR plays a critical role in MSC osteogenic differentiation and skeletal homeostasis and that dysregulation of p75NTR leads to postnatal skeletal defects. New findings from our studies may identify a novel factor that regulates osteogenesis, thereby presenting a promising therapeutic target for osteoporosis.
Associate Professor, Orofacial Sciences
Research interests: Biological processes underlying craniofacial bone regeneration, orthodontic appliances and techniques, molecular mechanisms of dental stem cells, osteoporosis, MSC-mediated craniofacial bone regeneration
Kazuhito Morioka, MD, PhD - Nociplastic spinal cord-muscle loop in degenerative disc disease (DDD)
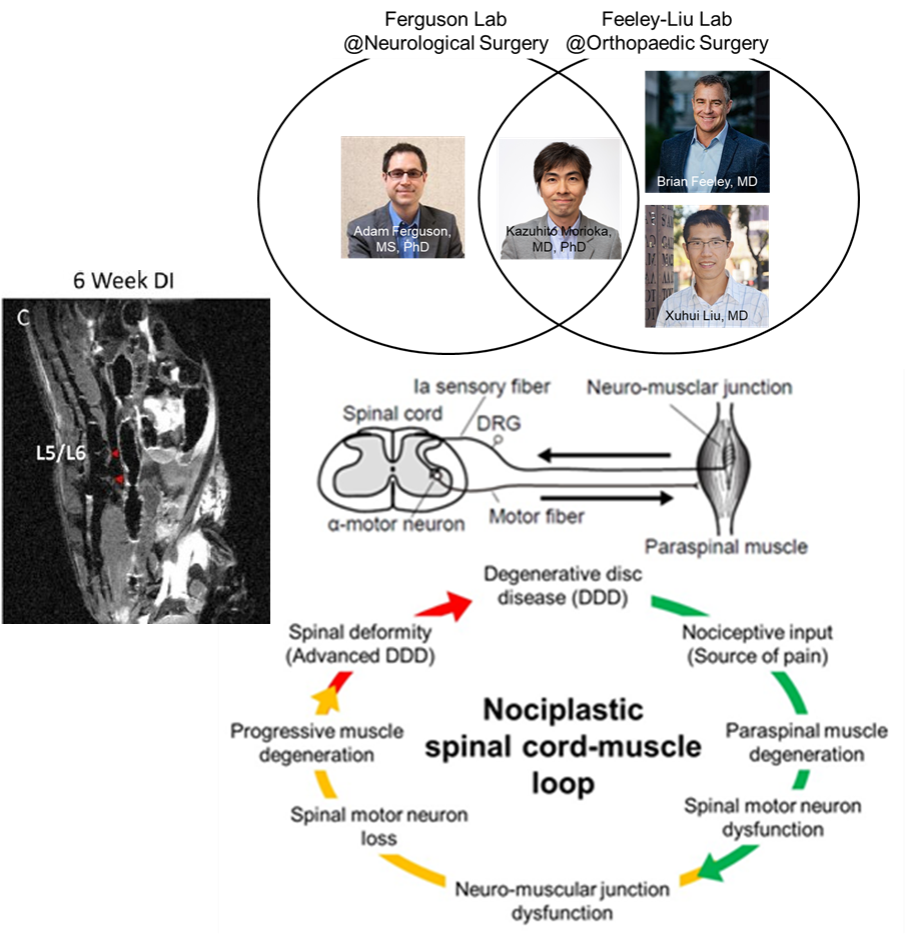
‘Nociplastic’ from “nociceptive plasticity” indicates a functional change in nociception due to increased sensitivity to pain in the central nervous system regardless of the obvious damage, disorder, or inflammation neurologically. Nociplastic changes play a role in the chronicity of pain resulting from the musculoskeletal system as well as paraspinal muscle degeneration due to the pain. However, the exact cause-effect relationships are still not fully characterized. From both neuronal and musculoskeletal perspectives, this proposed study aims to elucidate the longitudinal nociplastic mechanism in degenerative disc disease (DDD) for accelerating research on low back pain (LBP) supported by the CCMBM Pilot and Feasibility Grant Program.
This study enables our interdisciplinary collaborative team from Dr. Adam Ferguson’s laboratory (Neurological Surgery) and Dr. Brian Feeley & Dr. Xuhui Liu's laboratory (Orthopaedic Surgery) to address the fundamental issues regarding degenerative spinal disorders by leveraging the strength and expertise of each lab, allowing a more rigorous and comprehensive approach. Using a mouse model of lumbar disc degeneration with paraspinal muscle degeneration, we are exploring nociplastic changes involving apoptosis and trophic factors in the spinal cord and paraspinal muscle to identify distinct degenerative features contributing to the progression of DDD by biochemical analysis, microscopic analysis, behavioral analysis, and multivariate analysis. Findings from this study will help foster a better understanding of the degenerative pathology throughout the acute and chronic phases of DDD.
Assistant Professor, Orthopaedic Surgery
Research interests: Central nervous system disorders (spinal cord injury, traumatic brain injury), musculoskeletal system disorders (bone fracture, bone pain, low back pain), and both system disorders (polytrauma, chronic low back pain)
Valentina Pedoia, PhD & Drew Lansdown, MD - Multi-Task Deep Learning to Develop Automatic Scrapular Shape Extraction from Clinical MR
This study aims to use deep learning to perform automatic scapula bone segmentation and to simultaneously synthetize CT-like images. With funding from the CCMBM Pilot and Feasibility Grant Program, the proposed study builds a novel translational platform to revolutionize shoulder MR images in research studies, but also is paradigm-shifting in that it may provide a first step towards a more quantitative approach to surgical planning and patient management.
Methodology Needed to Obtain Bony and Soft Tissue Information
Scapular bone shape is an important determinant in surgical planning and predictor of post-operative outcomes for patients with shoulder instability and shoulder osteoarthritis. Currently, clinical evaluation of scapular bone shape is performed on a three-dimensional (3D) computed tomography (CT) scan, while a magnetic resonance imaging (MRI) scan is obtained to evaluate the soft tissue surrounding the shoulder. There is a clear clinical and research need for a methodology to obtain bony and soft tissue information from a single imaging study in an accurate, repeatable and fully automated fashion.
Deep Learning Allows Automated Segmentation of Cartilage and Bone
Deep Learning (DL), especially convolutional neural networks (CNNs), has made strides in several domains as speech recognition, visual object detection, classification, drug discovery and genomics. DL shines when afforded large datasets, as its automated feature extraction allows one to solve problems too complex for conventional approaches. CNNs are representation learning methods characterized by the usage of multiple, simple, but non-linear units to build several interconnected layers. Each layer aggregates the information at increasing levels of abstraction starting with simple image elements, as edges or contrast, to more complex and semantic aggregations, uncovering latent patterns able to accomplish pattern recognition tasks. DL has allowed for the automatic segmentation of knee cartilage, knee and hip bone; however, the scapula is a unique thin structure with a complex shape that poses different challenges.
Novel Directions for Shoulder Instability and Should Osteoarthritis in Clinical Treatment and Research
We have performed a pilot study utilizing a two-dimensional (2D) V-net convolutional neural network architecture with good results (mean Dice score coefficient: 82%), however further improvement is necessary for clinical or research implementation. We propose applying mixed precision training to allow for 3D processing in an efficient fashion. We also plan to augment our dataset with Statistical Shape Modeling to generate multiple synthetic training examples to be used for model pre-training and transfer learning. Finally, we plan to apply multi-task learning to simultaneously synthesize CT images and segment scapular bone from a standard clinical MRI scan. We will compare model performance on the MRI scan relative to a matched 3D-CT scan from previously acquired patient scans. The results of this study have the potential to greatly impact clinical treatment and create novel directions of research on shoulder instability and shoulder osteoarthritis.
Assistant Professor
Department of Radiology and Biomedical Imaging
Research interests: Medical imaging, computer vision, machine learning, big data analysis, MRI, musculoskeletal imaging, clinically oriented quantitative imaging, articular cartilage compositional imaging
Assistant Professor
Department of Orthopaedic Surgery
Research interests: Musculoskeletal quantitative imaging, sports medicine, ligament imaging, muscle imaging
Janet Lee, MD, MPH, MAS - Skeletal Effects of Puberty Suppression of Transgender Youth
Janet Lee, MD, MPH, MAS
Skeletal Effects of Puberty Suppression of Transgender Youth
Skeletal Effects of Pubertal Suppression in Transgender Youth (SEPSITY) is a 1-year collaborative pilot study investigating bone measures of early pubertal transgender and gender diverse (TGD) youth prior to and during gender-affirming medical therapy with gonadotropin-releasing hormone agonists (GnRHa). Data from Europe have shown that late pubertal and adult transfeminine individuals, in particular, have low BMD prior to and following several years of gender-affirming sex hormones. We have shown that early pubertal TGD youth of both designated sexes at birth also have higher than expected rates of low BMD by DXA and QCT prior to initiation of gender-affirming medical therapy, and that those with low BMD have lower physical activity than those with normal BMD.
From the UCSF Child and Adolescent Gender Center, we are building a cohort of TGD youth initiating gender-affirming medical therapy in early puberty, with plans to continue following these youth into adulthood. Study participants complete standardized assessments of bone mineral density (BMD) and body composition with dual-energy X-ray absorptiometry (DXA), bone microarchitecture with high-resolution peripheral quantitative computed tomography (HR-pQCT), strength measures, anthropometrics, bone turnover markers, vitamin D status, dietary calcium intake, and physical activity. Our pilot data are expected to lead to longer-term intervention studies aiming to mitigate the expected lag in skeletal development during pubertal suppression, and to identify potential areas for intervention. Ultimately, our research will positively contribute to the clinical care of TGD youth. Funding from the CCMBM pilot and feasibility award has allowed us to study and build this important cohort.
Janet Lee, MD
Assistant Professor, Pediatrics
Research Interests: Transgender health and bone, puberty and bone, puberty suppression and bone, cross hormone therapy and bone.
Jeannie Bailey, PhD - The mechanistic pathophysiology associated with paraspinal muscular degeneration and chronic low back pain
Jeannie Bailey, PhD
The mechanistic pathophysiology associated with paraspinal muscular degeneration and chronic low back pain
In this pilot study, we will assess the spatial distribution of multifidus fat infiltration and cellular-makeup of muscle biopsies from distinct groups of chronic low back patients to decipher the underlying mechanisms negatively affecting multifidus and its regenerative potential.
Chronic low back pain (CLBP) is the world's leading debilitating condition. The multifaceted and uncertain etiology underlying CLBP makes it notoriously difficult to determine specific diagnoses and effective treatments. The paraspinal muscles, particularly the multifidus (MF), have a uniquely important biomechanical role in stabilizing the lumbar spine and could be an effective target for conservative therapy. However, based on standard MRIs, MF muscle health is not shown to consistently associate with CLBP. MF is likely a critical component modulating the relationship between existing spinal pathology and patient symptoms, but the mechanisms underlying how MF is affected by spinal pathology remains unclear. We propose utilizing advanced MRI to precisely quantify fat infiltration spatial distribution, paired with knowledge of the underlying cellular composition and gene expression from corresponding MF tissue samples to clarify the cellular mechanisms compromising MF muscle quality. We will assess MF muscle quality and regenerative potential from two patient groups: a radicular CLBP group with disc herniation (n=8) and a localized CLBP group with disc pathology (n=8). First, using advanced MRI sequences for fat infiltration, we will distinguish patterns of relative quantity and spatial distribution of fat infiltration within MF between radicular and localized CLBP patient groups. Next, we will collect MF muscle tissue samples and analyze tissue-level MF composition based on presence and distribution of fiber type, fibrosis, fat cells, innervation, and inflammatory biomarkers. This will help decipher mechanisms affecting MF based on the diverse underlying cellular composition. Lastly, we will quantify the number of underlying fibro-adipogenic progenitor stem cells (FAPs) and use gene expression to determine cellular stemness, myogenesis, fibrosis, different adipose tissue types, inflammation, and innervation. This study will provide pilot data for us to pursue funding for a larger study. The goal of this overall work is have a clearer understanding of the pathophysiology of MF muscle degeneration and recovery potential associated with CLBP, which can lead to the development of therapies for muscle regeneration and targeted rehabilitation supporting focused conservative care approaches for managing and alleviating CLBP.
Jeannie Bailey, PhD
Assistant Professor, Orthopaedic Surgery
Research interests: Biomechanics and age-related conditions of the lumbar spine and participate in events and leverage collaboration between the departments of Orthopaedic Surgery and Physical Therapy, as well as utilizing Imaging and Epi biostats research cores.
Chelsea Bahney, PhD - Building a Better Bone: Promoting Endochondral Ossification to Stimulate Vascularized Bone Regeneration
Chelsea Bahney, PhD
Building a Better Bone:
Promoting Endochondral Ossification to Stimulate Vascularized Bone Regeneration
My research focuses on developing therapeutic strategies that recapitulate the normal sequence of fracture repair to improve clinical outcomes in bone regeneration. Cartilage is the natural precursor of bone during embryonic development, limb growth, and fracture repair. However, current therapies to treat challenging bone defects or stimulate de novo bone regeneration transplant bone to promote the process of intramembranous ossification. This approach fails to stimulate adequate angiogenesis within the bone graft, resulting in limited osteogenesis, poor osseointegration, and ostenecrosis: as a result clinical failure rates are estimated between 16-35%.
The conceptual foundation of my laboratory is that we can improve vascularized bone regeneration by using tissue engineering strategies to promote endochondral ossification. We propose engineering a cartilage template that will stimulate angiogenesis and osteogenesis for improved clinical outcomes in bone regeneration. Our preliminary pre-clinical studies have validated this approach and demonstrated that cartilage promotes a highly vascularized and integrated bone regenerate that outperforms allograft and is not significantly different than the gold standard bone allograft.1 (FIG 1)
The research efforts supported by the CCMBM Pilot grant aim to translate these preclinical data by developing an endochondral cartilage graft appropriate for human use. Using the CCMBM core facilities we are using novel analytical tools to understand how bioactivity of different types of cartilage vary and how to optimize this tissue for a safe and efficacious cartilage allograft. This research aims to understand how the extracellular matrix of cartilage plays a role in regulating either a stable articular phenotype that can be used for articular cartilage repair, compared to cartilages that stimulate bone formation.
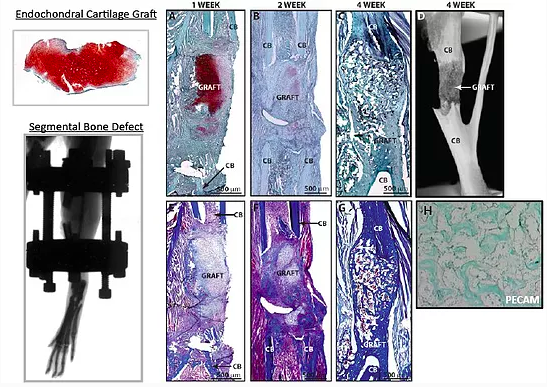
Endochondral cartilage grafts were isolated from the cartilage phase of fracture callus (top) and transplanted into critical sized segmental defect in an externally stabilized murine tibia (bottom). (A-C) Safranin-O staining shows loss of cartilage, while (E-G) Masson’s Trichrome staining demonstrates conversion to a traeculated bone state during the time course of healing (A&E) 7, (B&F) 14, or (C&G). (D) µCT image of tibia defect 4 weeks post-surgically demonstrates integrated bone formation. (H) PECAM staining indicates extensive re-vascularization in the graft. “cb” = cortical bone (host), “graft” = transplanted fracture callus cartilage.
LITERATURE CITED
1 Bahney CS, Hu DP, Taylor AJ, Ferro F, Britz HM, Hallgrimsson B, Johnstone B, Miclau T, Marcucio RS. (2013) Stem cell derived endochondral cartilage stimulates bone healing by tissue transformation. J Bone Miner Res. Epub 2013 Nov 21. Doi: 10.1002/jbmr.2148; PMID: 24259230
Chelsea
Assistant Professor, Orthopaedic Surgery
Research Interests: Endochondral bone regeneration, tissue engineering, polytrauma, chondrocyte transformation, stem cell therapies.
Xiojuan Li, PhD & Benjamin Ma, MD - In search of preventative measures for post-traumatic osteoarthritis: Synovial fluid characterization after anterior cruciate ligament injuries using novel techniques and approaces
Xiaojuan Li, PhD and Benjamin Ma, MD
In search of preventive measures for post-traumatic osteoarthritis: Synovial fluid characterization after anterior cruciate ligament injuries using novel techniques and approaches
The research efforts supported by the CCMBM Pilot grant aim to translate these preclinical data by developing an endochondral cartilage graft appropriate for human use. Using the CCMBM core facilities we are using novel analytical tools to understand how bioactivity of different types of cartilage vary and how to optimize this tissue for a safe and efficacious cartilage allograft. This research aims to understand how the extracellular matrix of cartilage plays a role in regulating either a stable particular phenotype that can be used for articular cartilage repair, compared to cartilages that stimulate bone formation.
We are exploring the role of inflammation on the development of posttraumatic osteoarthritis after ACL injury and reconstruction. Synovial fluid from these patients might tell us what inflammation does to the knee joint years after surgery.
We are following a cohort of patients who were recruited at the time of their acute ACL injury and are now 2-3 years out from ACL reconstruction. We have collected a myriad of data at multiple time points: biomechanics, quantitative MR, and 3D motion analysis, to name a few.
Our present topic focuses on synovial fluid drawn from these patients at the time of their ACL reconstruction. The biochemical profiles of synovial fluid can tell us great details about the cartilage as the body attempts to heal from injury. The inflammatory process at the time of injury has been proposed as a possible contributor to rapid cartilage degeneration that may lead to posttraumatic osteoarthritis. Our preliminary data shows high concentrations of cytokines and markers of cartilage turnover after injury. Some of these seem to correspond to synovitis on MRI and T1ρ relaxation times in the cartilage.
Synovial fluid NMR spectra show us another way of measuring inflammation and degradation by characterizing the metabolic profiles of tissues. Advantages of NMR techniques include the need for small amounts of sample for analysis (20ul), its non-destructive nature, and its high throughput without the need for pre-selection of analytical parameters or sample derivatization procedures.
Xiaojuan Li, PhD
Professor, Radiology
Research Interests: Medical imaging, MRI, MR spectroscopic imaging, clinically oriented quantitative imaging, cartilage degeneration, osteoarthritis, joint injury, rheumatoid arthritis, marrow adiposity and osteoporosis; MR techniques, musculoskeletal applications, arthritis
Benjamin Ma, MD
Professor, Orthopaedic Surgery
Research Interests: Non-invasive monitoring of joint condition, biomarkers, joint fluid assays & analysis, correlation of objective biomarkers with patient-reported outcomes.
Aaron Fields, PhD - Endplate dysfunction in disc degeneration: Investigating solute transport kinetics, disc cell viability, and new imaging methods
Aaron Fields, PhD
Endplate dysfunction in disc degeneration:
Investigating solute transport kinetics, disc cell viability, and new imaging methods
Why do some discs degenerate while others don’t? Knowledge from this research will advance our understanding of disc degeneration etiology and will help guide new prevention strategies, diagnostics and therapeutics. My broad research interests relate to structure-function relationships in musculoskeletal tissues. One specific focus is the cartilage endplate (CEP), which is a thin layer of cartilage that separates the intervertebral disc cells from their nutrient supply. Decline in nutrient supply is believed to be an important reason why disc cells fail to remodel their matrix. With support from the CCMBM Pilot and Feasibility grant program, we’re combining advanced microscopy techniques, new clinical imaging tools and a novel diffusion chamber to establish the effects of CEP structure on nutrient transport and disc cell function (Figure). The long-term hypothesis of this work is that a new MR imaging sequence that we developed to non-invasively assess CEP permeability can forecast which discs will degenerate and which will benefit from treatment. For this work, I’m collaborating with Dr. Roland Krug (Department of Radiology and Biomedical Imaging), an expert on MR pulse sequence development for musculoskeletal tissues.
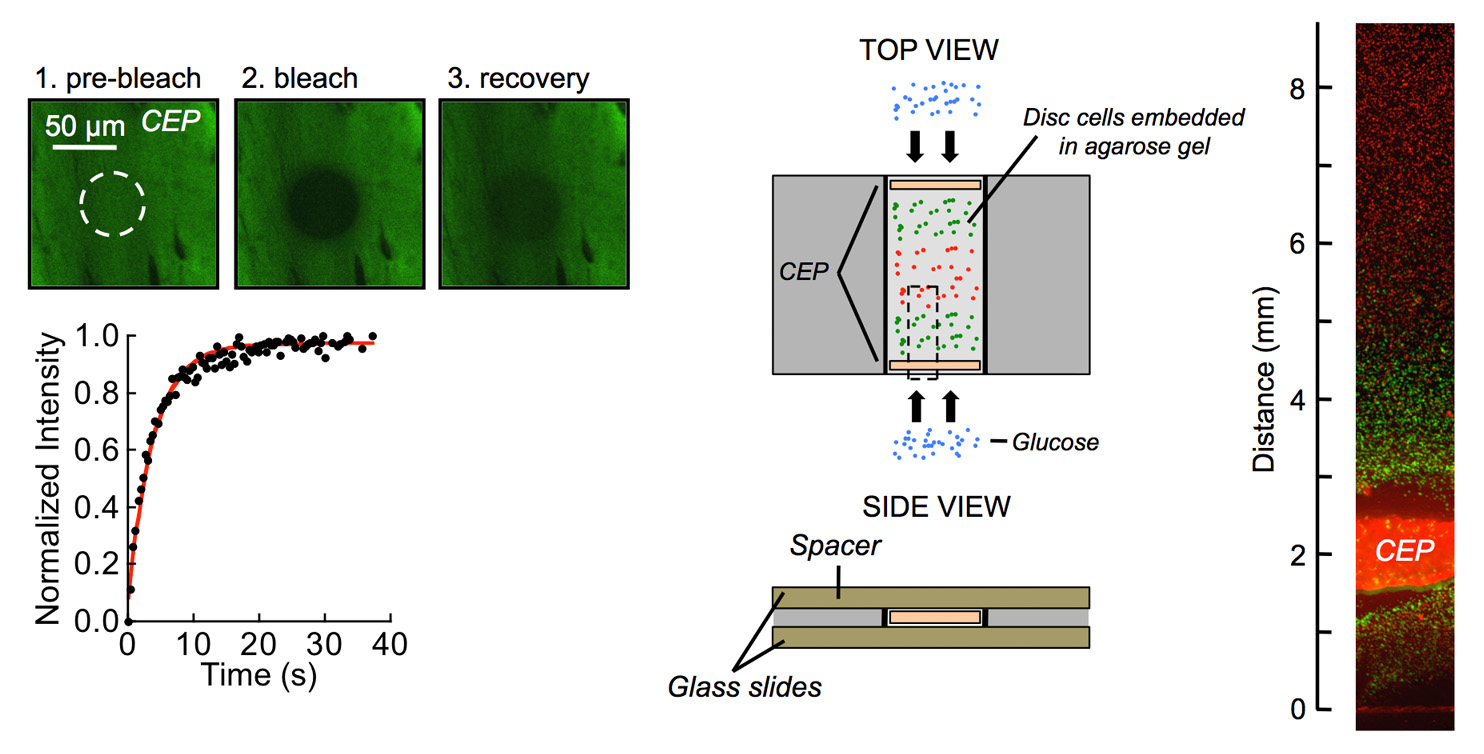
Aaron Fields, PhD
Assistant Professor, Orthopaedic Surgery
Research Interests: spine, biomechanics, intervertebral disc degeneration, osteoporosis, low back pain, finite element analysis.
Xuhui Liu, MD - Neuro-regulation of osteoporosis and heterotpic ossification after spinal cord injury
Xuhui Liu, MD
Neuro-regulation of osteoporosis and heterotopic ossification after spinal cord injury
The long-term goal of this research project is to understand the mechanism of central nerve system regulating skeletal system health. My research focuses on the molecular mechanisms of skeletal muscle atrophy, degradation and heterotopic ossification after direct and indirect injuries. My research focuses on key molecular pathways involved in skeletal muscle atrophy, fibrosis and fat infiltration, including Akt/mTOR, TGFβ and PPARg pathways. A significant part of my research program focuses on the transcriptional and post-transcriptional regulation of ECM
Xuhui Liu, MD
Associate Professor, Orthopaedic Surgery
Research Interests: Atrophy, skeletal muscle, matrix metalloproteinases, gene transcription regulation, ligament and tendon, rotator cuff, articular cartilage, muscle fat infiltration, mesenchymal stem cell, heterotrophic ossification, osteoporosis.